11th August, 2021
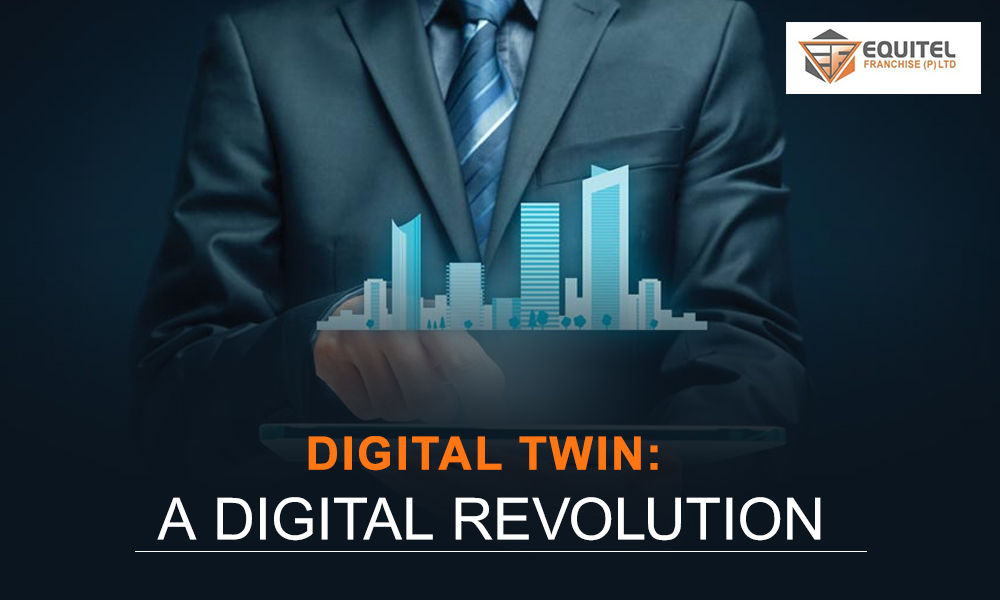
Even if all transformers are of the same specifications, each becomes a unique entity to be cared for, especially when it ages. Digital Twin maps each transformer in the substation. The Digital Twin of a power transformer can also be used for controlling the actuator.
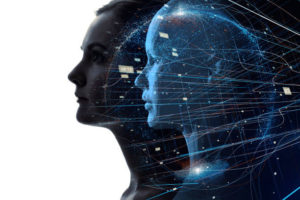
The increasing digitalization of assets is resulting in an avalanche of data. The knowledge of these assets could be leveraged for making better-informed decisions. When the present grid moves more towards the smart grid, a more pervasiveness of information and communication technology could be expected. The increasing penetration of intermittent renewable energy sources and incorporation of utility-scale energy storage systems in the near future and technologies like electric vehicles adds in more uncertainties in the energy grid. The power transformers in the grid assume a very significant role in the transmission and distribution of power. A failure of which could result in catastrophic consequences in terms of stability of the grid and its economic implications. The modern grid is not a mere interconnection of energy devices, it is also a network of measurement devices and communication such as Supervisory Control And Data Acquisition (SCADA).
IoT and big data
According to a survey published by the International Council on Large Electric Systems (CIGRE) one of the major failures of the transformer was related to insulation breakdowns. The transformer condition is monitored using oil tests, temperature measurements and onsite inspections. Various tests are in place for testing power transformer while it is in operation –like Dissolved Gas Analysis (DGA), partial discharge detector, Furfuraldehyde Analysis (FFA), temperature measurements, thermal imaging, etc., many of which are available for online monitoring of transformers as discrete units as well as integrated solutions. With the expansion of the Industrial Internet of Things (IIoT), the addition of more such online monitoring systems becomes obvious.
Digital Twins of transformers
Internet of Things (IoT) is an interconnection of physical ‘things’ that can interact with its physical environment with sensors/actuators along with computing and communication capabilities to exchange data with the internet. The IoT devices have become ubiquitous in consumer electronics with the rise of smartphones, wearable health monitors, home automation controllers, etc. IoT in the context of the industrial environment is termed as Industrial Internet of Things (IIoT). The increased acceptance of smart grid technologies accelerates the deployment of IIoT in power systems, especially in the advanced metering infrastructure, digital protection systems, phasor measurement units, intelligent electronic devices, and asset monitoring systems. These produce an enormous amount of data in the power system, which could be overwhelming for traditional decision-making approaches. When considering an example of a 50 Hz three-phase voltage signal sampled at 128 samples per cycle, generates a dataset of 19200 samples per second. The collection of data, which is characterized by massive volume, high velocity, and heterogeneous variety is termed big data. The data from the big data analytics are applied to make sense of the collected data.
The concept of Digital Twin was introduced in a presentation related to product life management by Dr. Michael Grieves of the University of Michigan in 2002. The name Digital Twin was put forth by John Vickers of NASA in 2010. The Digital Twin encompasses the physical system in the real world, its digital model, and the communication linking them both. The digital thread concept forms the basic subunit of a Digital Twin, which is essentially the information that could trace back to the real-world system. The expansion in the Digital Twin is fuelled by the Internet of Things (IoT) and reducing costs of computational and storage resources. The data from various sensors are aggregated by the monitoring system. The raw data collected from the sensors along with additional features when used with appropriate machine learning algorithms could be used to create a more realistic model of a real physical asset. A Digital Twin goes a step further to keep the digital footprint of the transformer throughout its lifetime. The data collected over time is analyzed for trends of relevant features. This enables Digital Twins to be used for condition monitoring, predictive maintenance, and remaining useful life estimation. Digital Twin creates a digital trace, so in case of a mishap, it can be investigated with the digital counterpart. The Digital Twin concept has been researched in various industry segments such as manufacturing, healthcare, driverless autonomous vehicles, and machine-to-machine interactions.
Digital Twins of transformers are virtual replicas of physical transformers, which engineers and consultants can use to run simulations and test scenarios before the actual devices are built, deployed, or operated. Digital Twin technology has moved beyond the manufacturing segment and into the merging worlds of the Internet of Things, Artificial Intelligence, and Data Analytics. Digital Twin collects data from sensors and analyses to initiate the response through actuators.
The Digital Twin may be deployed either on a cloud platform or an edge computing device. The Digital Twin deployed on the cloud platform can take advantage of higher computing resources and big data analytics. A Digital Twin on edge may be used for quick dynamic decisions and controls in order to avoid any latency in communication channels and information processing. Also, the edge-based solution is called for when considering privacy and information security. So, one of the strategies is to have an edge model for quick decisions and computationally demanding analytics on cloud.
Asset management and Predictive maintenance using Digital Twin
The Digital Twin concept provides a great choice for modeling complex systems such as a power transformer. A Digital Twin of a transformer is a virtual image of the real-world transformer, which captures its historical, static and dynamic characteristics. Any changes in the actual transformer parameters get reflected in its digital counterpart. The time-based variation of various parameters of the transformer need to be captured as transformer health is influenced by aging and maintenance operation. The model is initially loaded with a set of scenarios and while in operation its measurements from the transformer are used as feedback to keep the model updated. The Digital Twin of the transformer once made can be used for simulating the power transformer in all different conditions.
One of the popular yardsticks for transformer health is the dissolved gas content in the transformer oil. Traditionally dissolved gas analysis is performed in an external lab by taking an oil sample, now an online DGA kit is being set up along with the transformer. The condition evaluation criterion based on the DGA results is mentioned in IEC 60599 and IEEE C57.104.
Several studies could be observed in the literature, which tries to estimate various transformer conditions based on DGA results. This includes traditional ratio methods, Duval’s Triangle method, and intelligent methods like fuzzy logic, Artificial Neutral Networks (ANN), Support Vector Machines (SVM), etc. The DGA in itself does not give a complete picture of the transformer condition. DGA results are just one among several measurements associated with a power transformer. The data generated from multiple sensors need to be collectively analyzed along with operational history to comprehend the condition of the transformer.
The monitoring device generates data continuously about the transformer under observation. This is apart from maintenance data, lab test results and equipment details. The Digital Twin keeps on building the digital thread of an asset right from its deployment, operation, and finally up to its termination. The magnitude of the data makes it difficult for humans to understand, but an appropriate algorithm could comprehend. In a substation with multiple transformers even if all transformers were of the same specifications, each becomes a unique entity to be cared for especially when it ages. So, each transformer in the substation would be mapped over to a unique Digital Twin. The operator can use the Digital Twin and its visualization real-time monitoring, situational awareness, stability analysis, planning, fault identification, schedule upcoming maintenance, and take better decisions. The Digital Twin of power transformers could also be used for controlling the actuators as power transformers are now being equipped with actuators like valves, cooling systems, dehumidifiers, tap changers, etc. The utility company may use its collection of Digital Twins of its fleet of power transformers for contingency analysis, maintenance schedule, and budget allocations.